Wildfire resilience
Community resilience to wildfires - A network analysis approach by utilizing human mobility data
Disasters have been a long-standing concern to societies at large. With growing attention being paid to resilient communities, such concern has been brought to the forefront of resilience studies. However, there is a wide variety of definitions with respect to resilience, and a precise definition has yet to emerge. Moreover, much work to date has often focused only on the immediate response to an event, thus investigating the resilience of an area over a prolonged period of time has remained largely unexplored. To overcome these issues, we propose a novel framework utilizing network analysis and concepts from disaster science (e.g., the resilience triangle) to quantify the long-term impacts of wildfires. Taking the Mendocino Complex and Camp wildfires - the largest and most deadly wildfires in California to date, respectively - as case studies, we capture the robustness and vulnerability of communities based on human mobility data from SafeGraph from 2018 to 2019. The results show that demographic and socioeconomic characteristics alone only partially capture community resilience, however, by leveraging human mobility data and network analysis techniques, we can enhance our understanding of resilience over space and time, providing a new lens to study disasters and their long-term impacts on society.
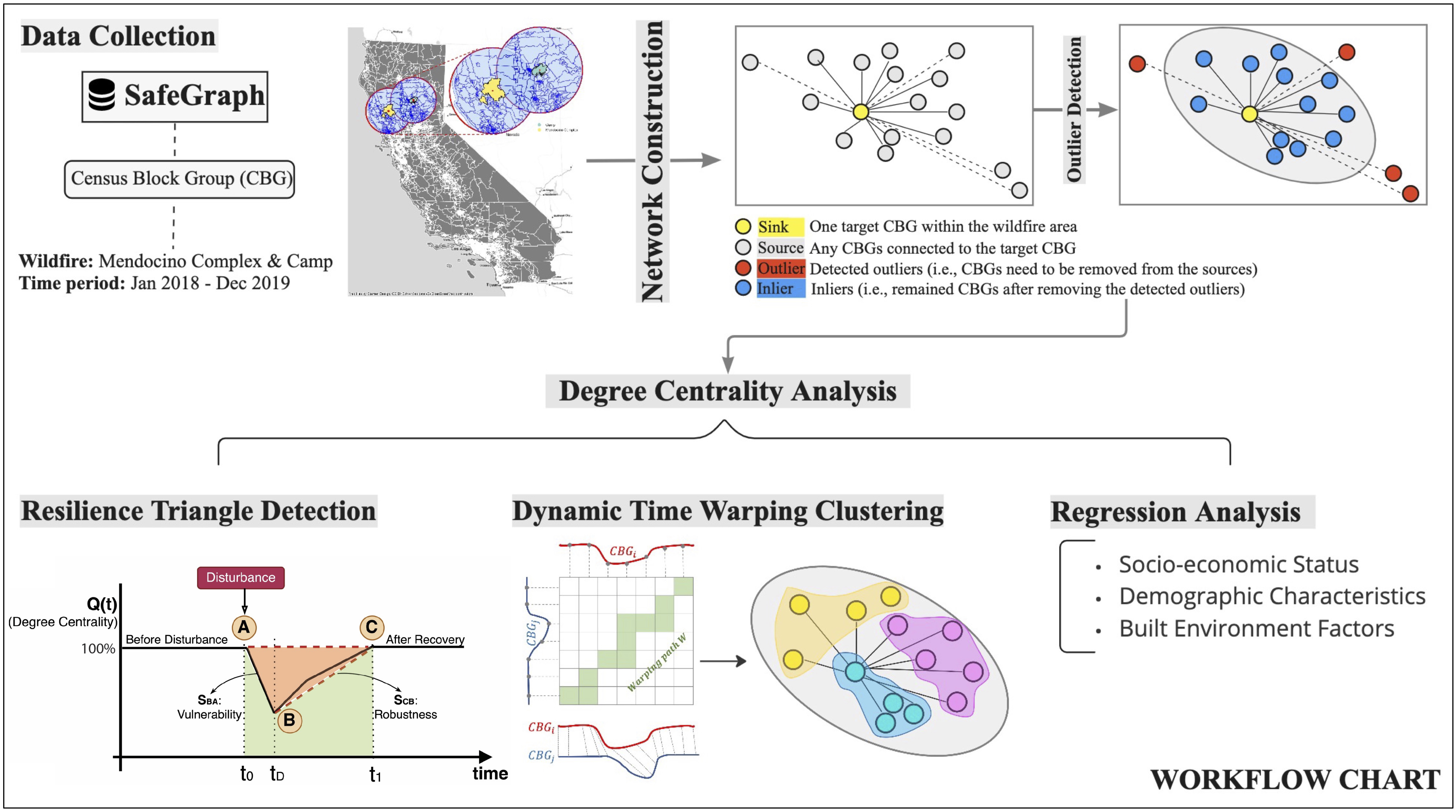
We borrows the concept of resilience triangle from disaster science to quantify resilience at the Census Block Group (CBG) level. The concept of resilience triangle was first introduced by Bruneau in 2003, to demonstrate a quantitative conceptual framework for measuring disaster resilience. In general, it can be used to measure the abrupt functionality loss of a social unit, like a community, over time under the disruption of a disaster. Besides, we also bring in the notion of degree centrality as a proxy for disaster resilience evaluation due to the consideration of the level of exposure of a CBG to a disaster. In other words, we take each CBG as a node and consider the connection between two CBGs as a link weighted by the frequency of visitation between the two. A node with high degree centrality indicates higher probability to be disrupted when being hit by a disaster. Therefore, we are able to identify the resilience triangle for each CBG.
Figure 2 gives two examples of detected traingles. The green dash line represents the pre-wildfire baseline level, which is defined as the two-month average degree centrality right before a disaster happens. It is considered as normal status and used to evaluate the recovery status. \(t_0\) is defined by the onset of a disaster, \(t_1\) is the time when the degree centrality reaches its minimum value between \(t_0\) and \(t_2\). \(t_2\) is the time when the degree centrally fully bounces back to the pre-wildfire baseline level, the green dash line aforementioned. However, if a CBG never fully restores to the baseline level, in this case, \(t_2\) is assigned by to the time when the maximum degree centrality occurs after the wildfire becomes inactive and we call this new normalcy.
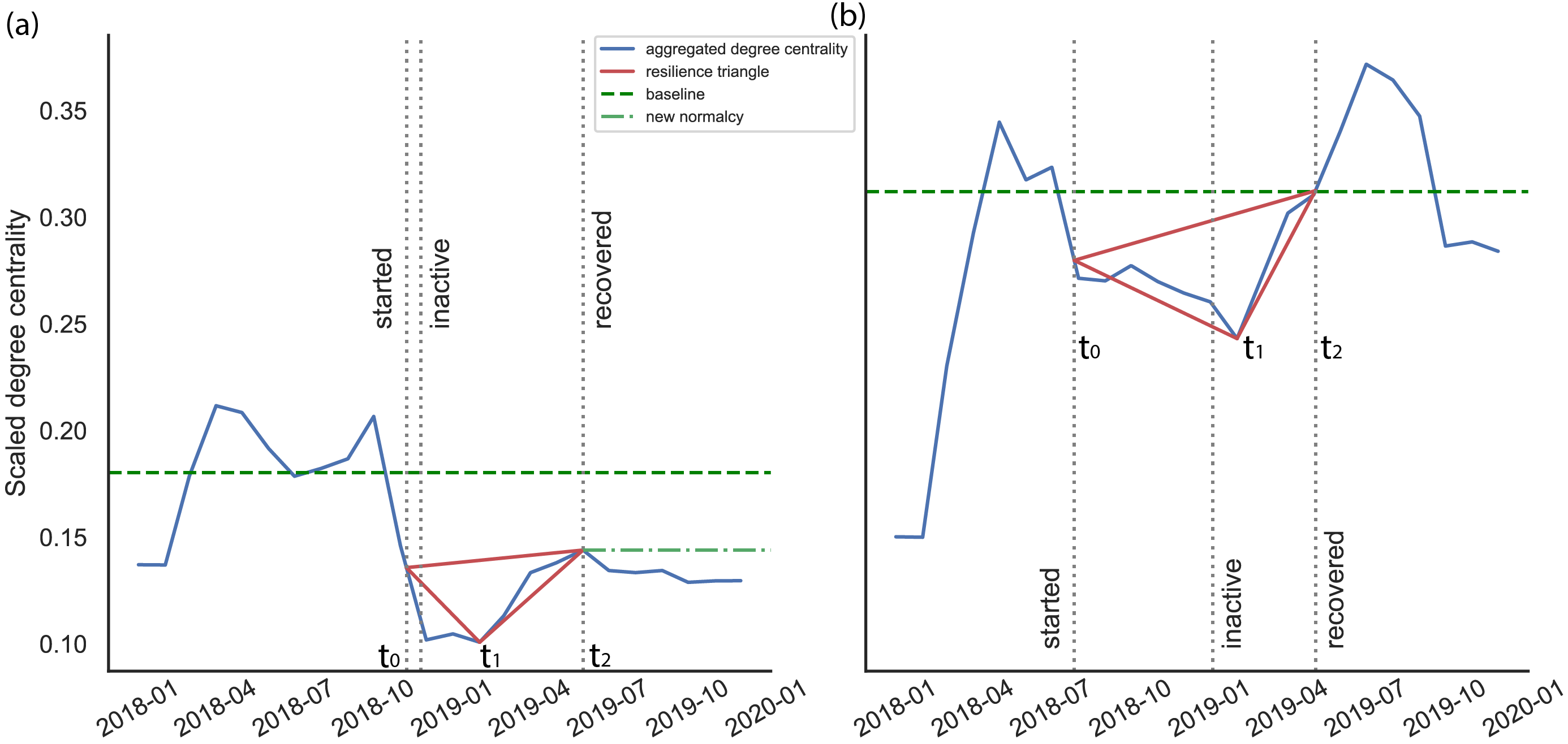
As there can be many CGBs within the wildfire areas, which makes the comparison between CBGs become difficult. To overcome this challenge, we used Dynamic Time Warping (DTW) clustering, which is known as an accurate method for clustering time series data, to classify CBGs based on their changing patterns of degree centrality. Figure 3 denibstrates the temporal distribution of the degree centrality for every CBG within each wildfire area from January 2018 to December 2019 colored by different clusters. As the MC and Camp wildfires started on August 2018 and November 2018, respectively, we observe a sharp decline in the degree centrality after the onset of the events, indicating the immediate impacts of disruptions caused by the wildfires on human movements. Afterward, the degree centrality continues to decrease and reaches its lowest point after a period of time. Then, it gradually bounces to its pre-wildfire status or establishes a new normalcy.
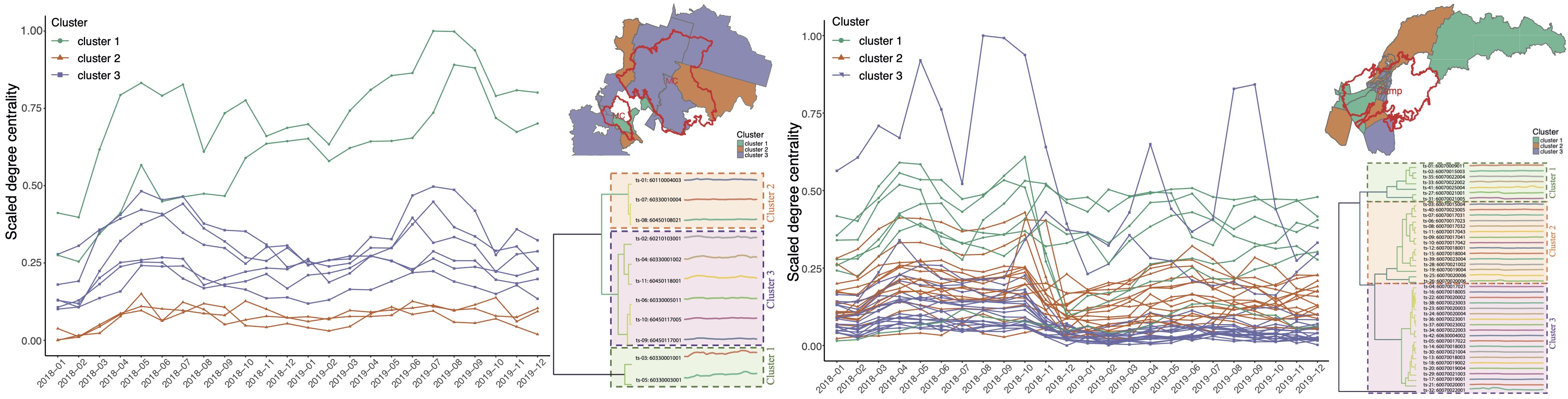
Figure 4 displays resilience triangles for each CBG (i.e., lines with transparent color) and the overall resilience triangles for the three clusters (i.e., triangles with non-transparent color). The resilience triangle records the abrupt losses in performance of a social unit (here referring to a CBG) under the disruption of a disaster. The depth of the triangle illustrates the severity of the disruption, the length of it demonstrate the recovery time, and the area of it indicates the resilience of the social unit. The smaller the area is, the more resilient the social unit is. By comparing the derived resilience triangles, we find that cluster 2 is most resilient cluster, followed by cluster 3 and cluster 1 in the Camp wildfire. In terms of the MC wildfire, cluster 1 is the most resilient, followed by the clusters 3 and 2.
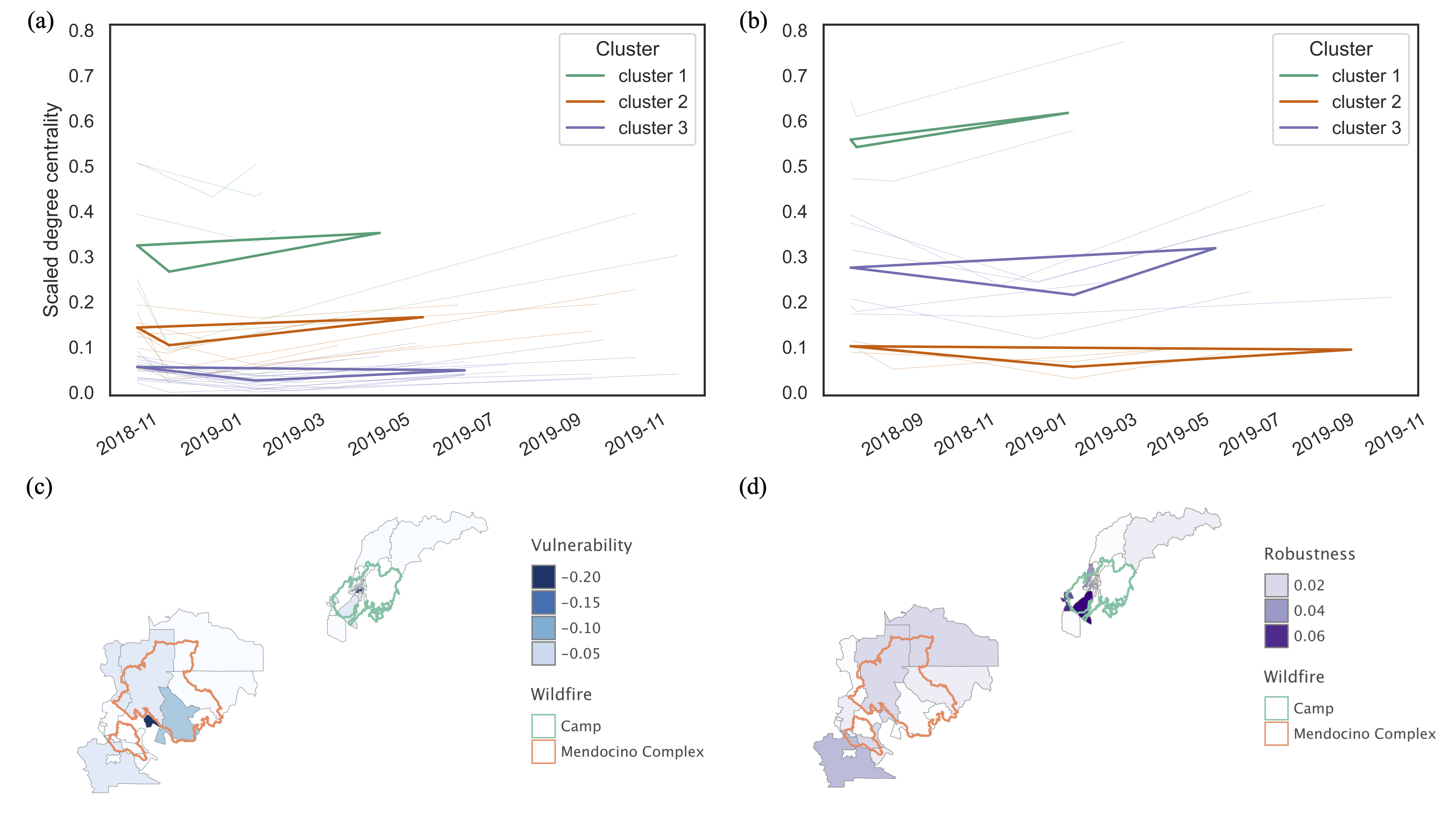
To explain the potential underlying factors related to these clusters, we conduct regression analysis for each wildfire. Table 1 gives a statistical summary of the outcomes of the regression models for the two wildfires. The result shows that the model identifies around 88% of variance of the degree centrality (Adjusted R2 = 0.8787) that is explained by the input variables. Further, most factors, except the number of full-time workers, percentage of people with degree higher than undergraduate, are highly correlated to the degree centrality. For the Camp wildfire, the model shows a relatively weaker ability to identify the variance of the degree centrality explained by the input variables (Adjusted R2 = 0.5832). One possible reason could be that there are much more observations (N = 950) than that in the MC wildfire (N = 288), which can therefore result in more noise in the model.
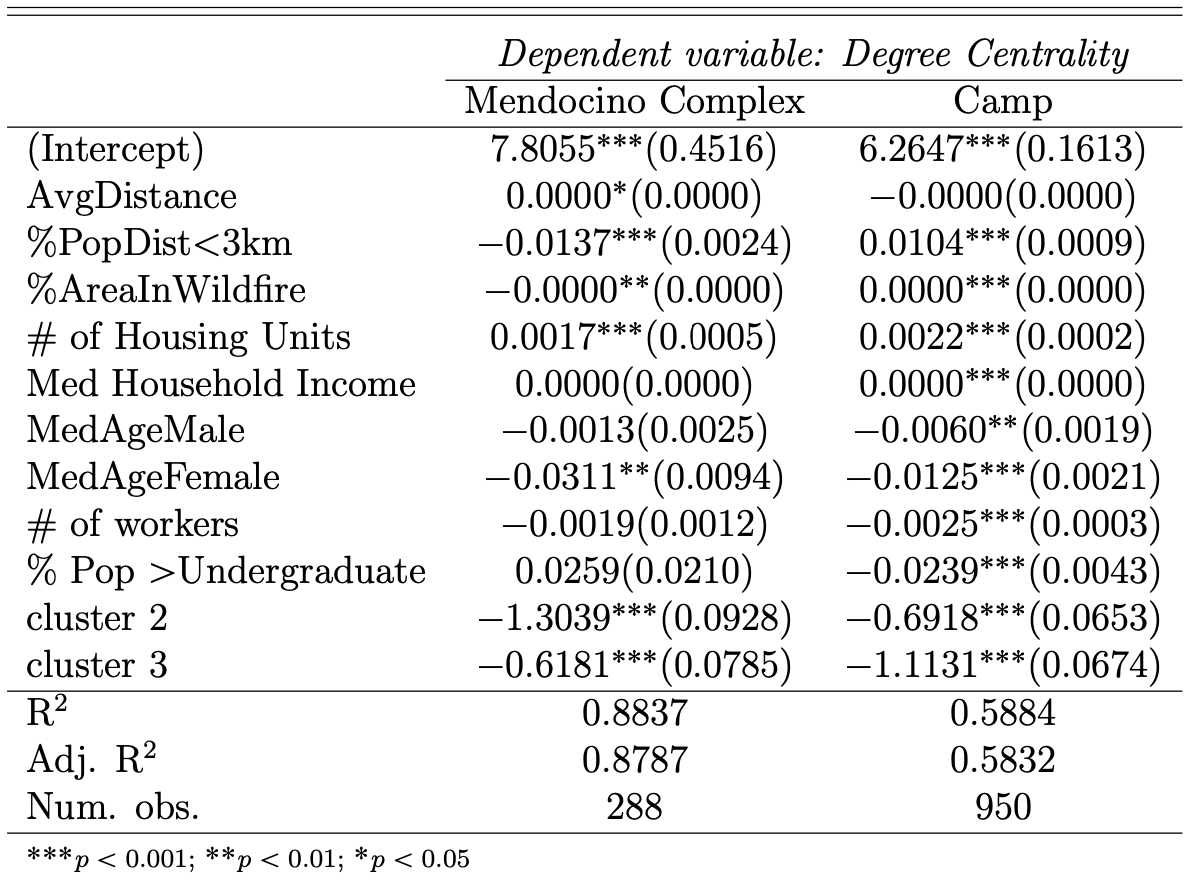
Highlights
- Quantifying community resilience is an open research challenge
- This paper develops a novel framework to quantify resilience after a disaster using network analysis
- Using human mobility data associated with two wildfires, we measure robustness and vulnerability of communities
- Results show community resilience is highly related to socio-economic and built environmental characteristics of the affected areas
- Our approach paves a way to study disasters and their long-term im- pacts on society
Chen, Q., Wang, B., Crooks, A. (2024). Community resilience to wildfires : A network analysis approach by utilizing human mobility data. Computers, Environment and Urban Systems, 110, 102110, https://doi.org/10.1016/j.compenvurb-sys.2024.102110.