Vaccination debate
Analyzing the vaccination debate in social media data Pre- and Post-COVID-19 pandemic
The COVID-19 virus has caused and continues to cause unprecedented impacts on the life trajectories of millions of people globally. Recently, to combat the transmission of the virus, vaccination campaigns around the world have become prevalent. However, while many see such campaigns as positive (e.g., protecting lives), others see them as negative (e.g., the side effects that are not fully understood scientifically), resulting in diverse sentiments towards vaccination campaigns. In addition, the diverse sentiments have seldom been systematically quantified let alone their dynamic changes over space and time. To shed light on this issue, we propose an approach to analyze vaccine sentiments in space and time by using supervised machine learning combined with word embedding techniques. Taking the United States as a test case, we utilize a Twitter dataset (approximately 11.7 million tweets) from January 2015 to July 2021 and measure and map vaccine sentiments (Pro-vaccine, Anti-vaccine, and Neutral) across the nation. In doing so, we can capture the heterogeneous public opinions within social media discussions regarding vaccination among states. Results show how positive sentiment in social media has a strong correlation with the actual vaccinated population. Furthermore, we introduce a simple ratio between Anti and Pro-vaccine as a proxy to quantify vaccine hesitancy and show how our results align with other traditional survey approaches. The proposed approach illustrates the potential to monitor the dynamics of vaccine opinion distribution online, which we hope, can be helpful to explain vaccination rates for the ongoing COVID-19 pandemic. Figure 1 displays an overview of the research outline.
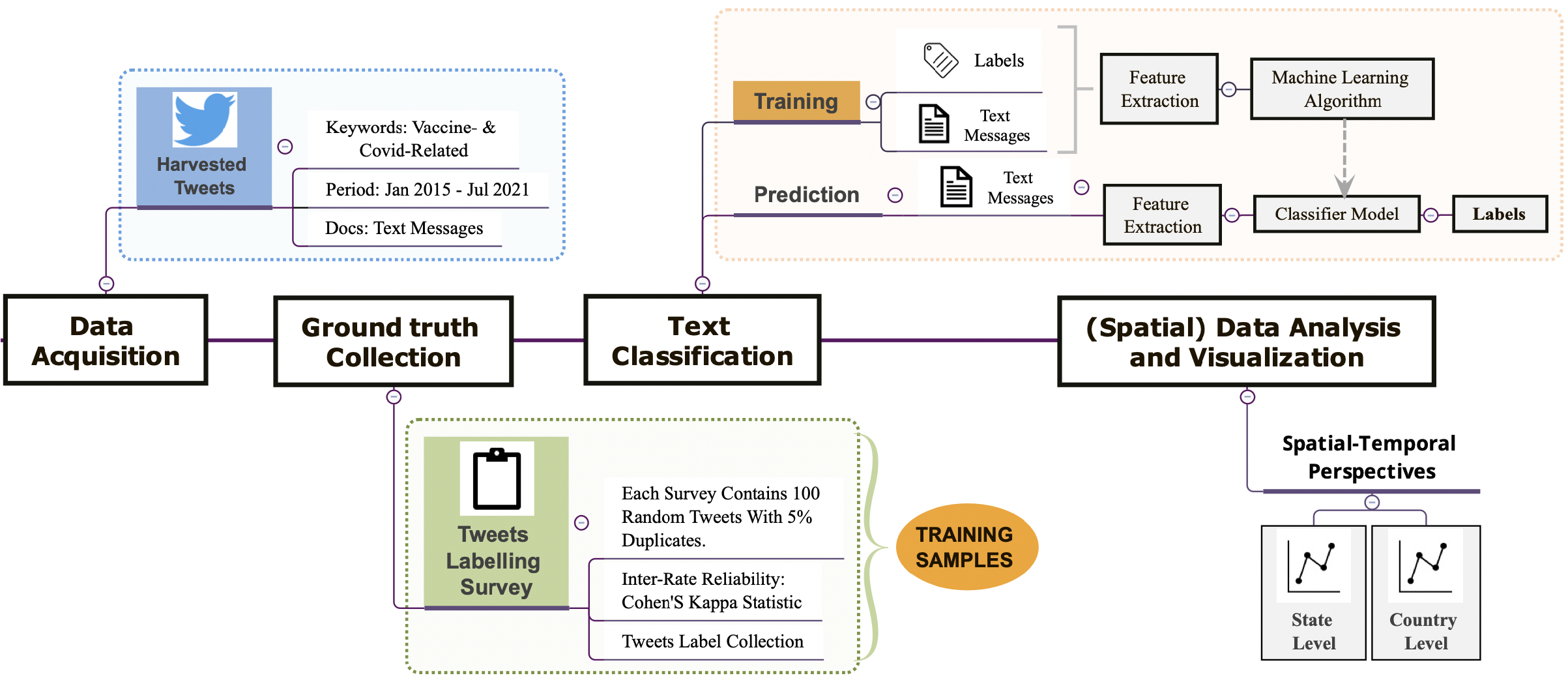
Text sentiment classification
To operationalize the proposed method, we first conducted a series of text cleaning, which generally relates to removing noise in the contents that do not contribute the classification process (e.g., multiple consecutive same characters, Unicode characters, hashtags, URLs, etc). Afterwards, we applied Word2Vec to convert words mathematically into a vector representation. The embeddings were then used as the input in a set of machine learning algorithms for classification, including Naive Bayes, Support Vector Machine (SVM), Logistic Regression, and Extreme Gradient Boosting (XGBoost) in order to see which one provides the best performance. To optimize the model performance, 5-fold Cross-Validation (CV) and hyperparameter tuning were applied. By comparing the performance on the test set, we found XGBoost stands out from other algorithms with an accuracy of 74%. Then, the XGBoost classifier was applied to detect the sentiments (i.e., Pro-vaccine, Anti-vaccine, Neutral) of each tweet in the rest data corpus.
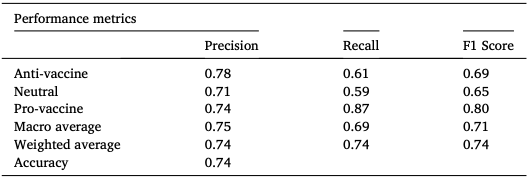
Vaccine hesitancy estimation
In addition to detecting the three sentiments (Pro-vaccine, Anti- vaccine, Neutral), we took one step further to analyze vaccine hesitancy. We proposed a metric called A2P Ratio (i.e., the ratio of Anti-vaccine to Pro-vaccine) as a simple proxy to quantify vaccine hesitancy due to its nuanced nature. The larger the ratio, the higher the vaccine hesitancy. To validate the proposed metric, we further compared it with the estimated vaccine hesitancy from CDC at the state level. By doing so, we are able to identify the potential correlation between the two, so as to use the “A2P Ratio” as a simple proxy to quantify vaccine hesitancy in a more efficient way. However, it is important to stress that the “A2P Ratio” introduced here only focuses on sentiment derived from the online vaccination discussions, and as such works as a simple alternative way to provide informed insights into vaccine hesitancy. Yet, to develop a more comprehensive understanding of vaccine hesitancy will require incorporating many other factors aforementioned, such as demographic, political, cultural factors which is beyond the scope of the current paper.
Results
Figure 3 shows the distribution of vaccination sentiment from 2015 to 2021 at state level. We can see that for most states, the rate of “Anti-vaccine” users increased in 2020 compared to 2019 and showed a minimal drop in 2021, while the changes in the rate of “Pro-vaccine” users over time are the opposite.
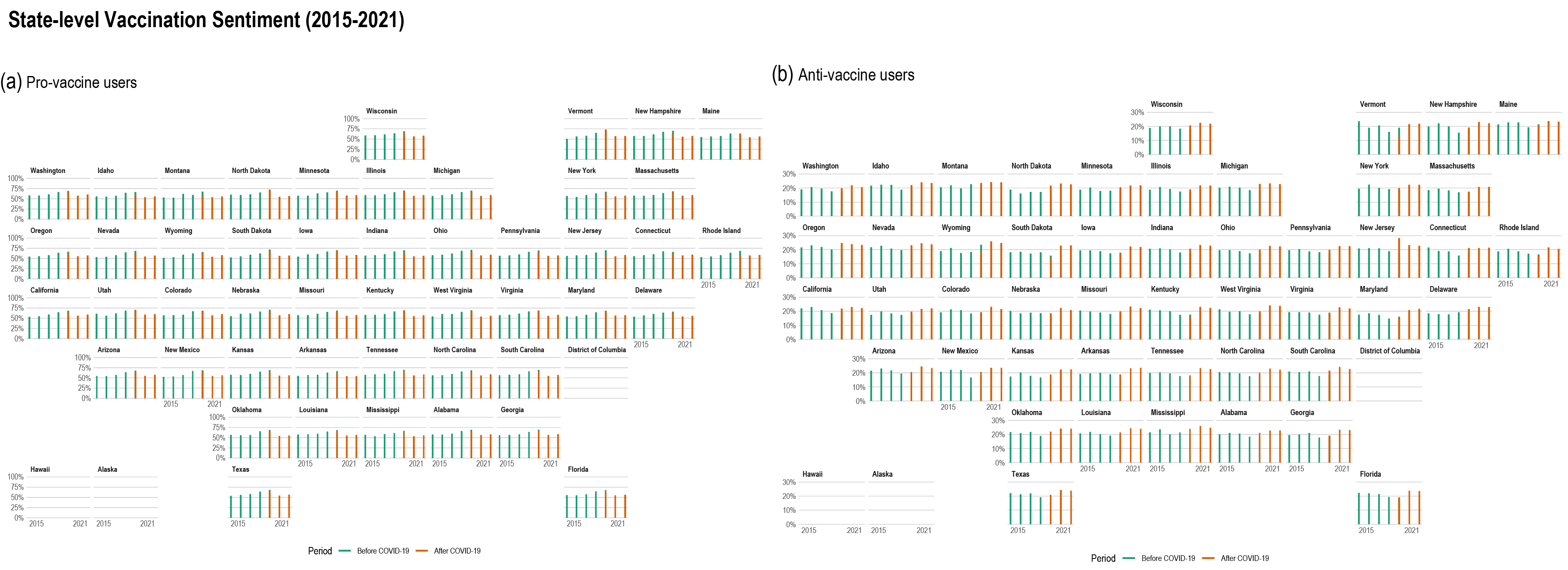
Moreover, in order to understand the potential correlation between the positive vaccine attitude online and the actual vaccination rate offline, especially after the COVID-19 outbreak, we compared the odds ratio of the Pro-vaccine users after the outbreak to that of the coronavirus vaccinations in each state.
\[OR_{\text{Pro-vaccine}} = \frac{\frac{N_{\text{Pro-vaccine users in a state}}}{N_{\text{Pro-vaccine users in the US}}}}{\frac{N_{\text{Twitter users in a state}}}{N_{\text{Twitter users in the US}}}}\]Figure 3 (a) & (b) display the spatial distribution of the odds ratios of the “Pro-vaccine” users and vaccination records, separately. The results reveal there was geographic difference in Pro-vaccine sentiment on Twitter. More specifically, states, such as Massachusetts (MA), Con- necticut (CT), Vermont (VT), Colorado (CO), Washington (WA), New York (NY), had relatively higher Pro-vaccine odds than other states (see Fig. 3 (a)). Part of the reason for this could be attributed to the relatively complete health system in these states as it has been shown that a well-functioning health system is crucial for improving vaccine coverage. Our finding follows a similar trend to that of the actual vaccination rate (see Fig. 3 (b)), which implies the positive attitude regarding vaccines identified from the social media data can, to some extent, reflect the actual vaccination rate offline. This was further validated by measuring the correlation coefficient between the two. Figure 3 (c) presents the correlation between the odds ratio of actual vaccination records and the odds ratio of Pro-vaccine users, where a positive correlation (R = 0.67, R2 = 0.45) between the two was observed. We argue that the proposed approach for identifying positive vaccine sentiments online can be used as an indicator for evaluating offline vacci- nation rates.
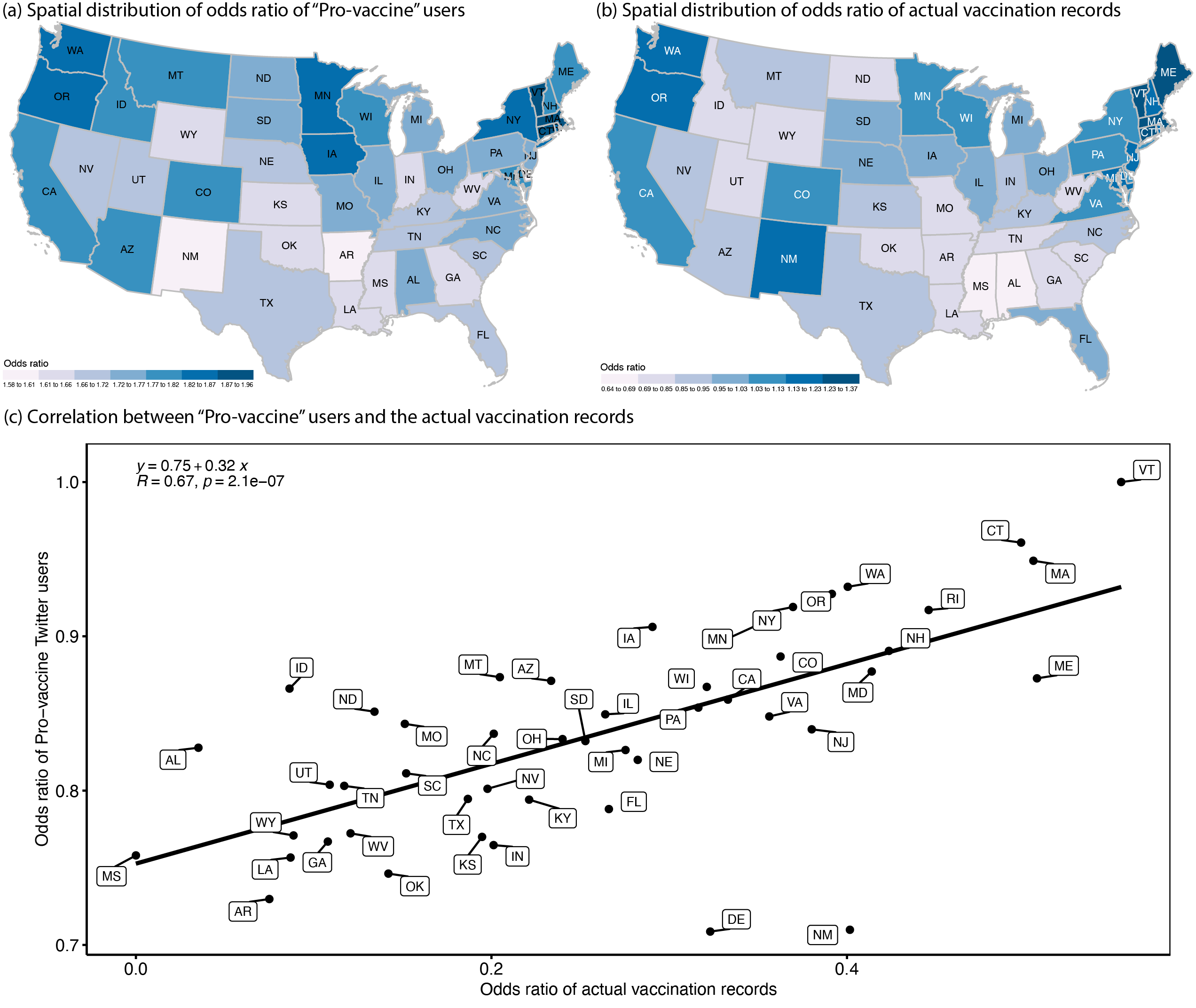
To demonstrate public’s attitudes identified from online vaccine discussion can be useful for predicting the tendency of vaccines hesitancy offline, we used the A2P Ratio as a proxy of vaccine hesitancy prediction and compared it with the estimated vaccine hesitancy rate from CDC, which is measured based on the U.S. Census Bureau’s Household Pulse Survey (HPS) (see Fig. 4). We observed that relatively higher A2P ratios and estimated vaccine hesitancy are mostly entrenched in states in the West and South, such as Wyoming (WY), Arkansas(AR), Florida (FL), Louisi- ana (LA), Nevada (NV), and so on. Besides this, we also observed that WY stood out from the other states in both maps, appearing as the most vaccine-hesitant state in the country. An important reason may be the inequality in resources allocation and distribution. Other possible reasons could be, for example, cultural conservatism, safety concerns, distrust of government, low health literacy, and so on. The results indicate the proposed A2P ratio has the ability to capture a comparable pattern as the estimated vaccine hesitancy obtained from a survey research. To quantified the relationship between the two, we conducted a correlation analysis and found they indeed had a positive correlation (R = 0.66, R2 = 0.43) as shown in Fig. 4. This finding implies the proposed A2P ratio can effectively estimate vaccine hesitancy, complementing the limitations of vaccine hesitancy based on survey research, such as difficulties in scaling up, time-consuming, and labor-intensive.
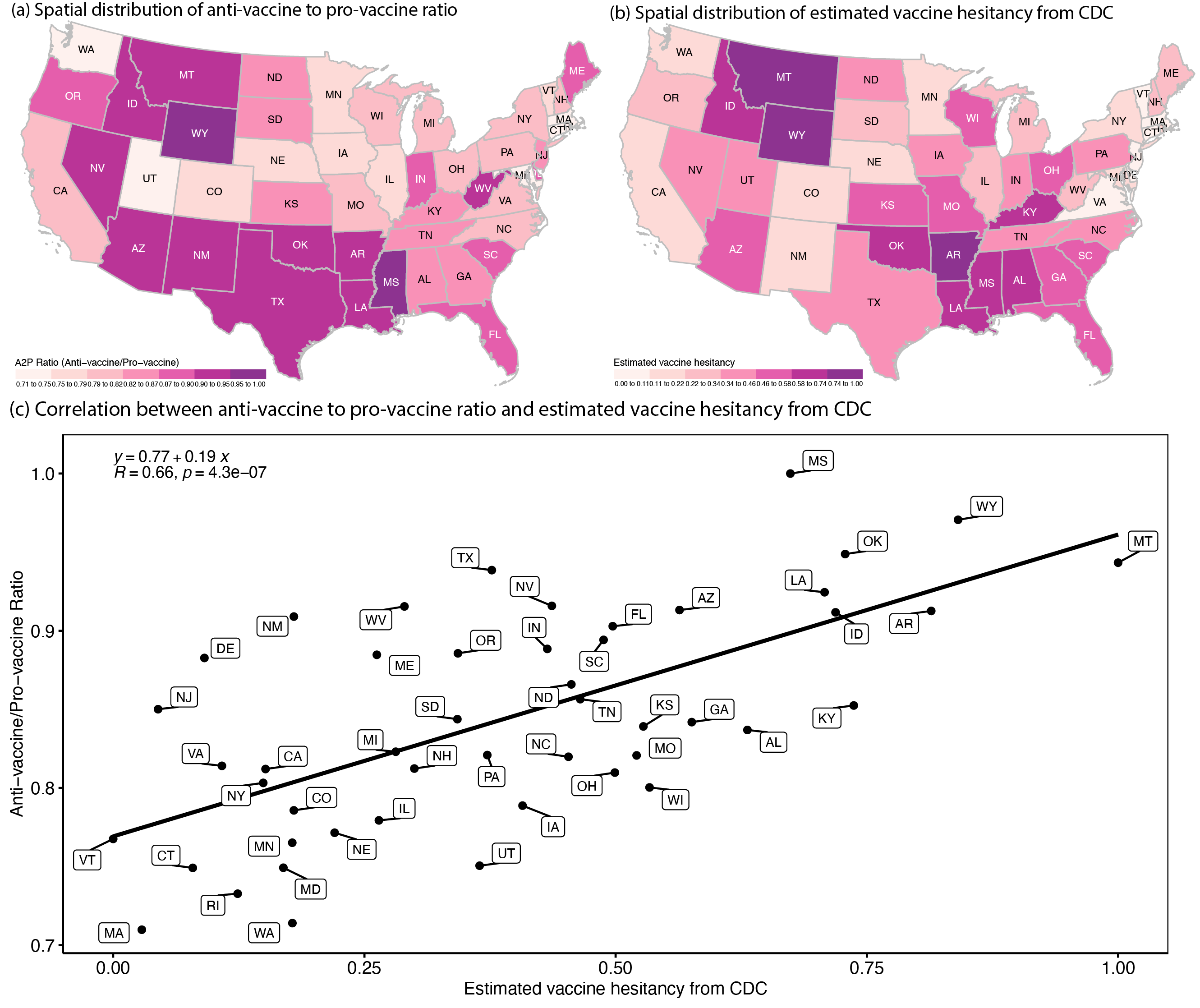
For more details about the project, please check out our paper below:
Chen, Q. and Crooks, A. (2022). “Analyzing the Vaccination Debate in Social Media Data Pre- and Post- COVID-19 Pandemic”, International Journal of Applied Earth Observation and Geoinformation. 110, 102783 https://doi.org/10.1016/j.jag.2022.102783